


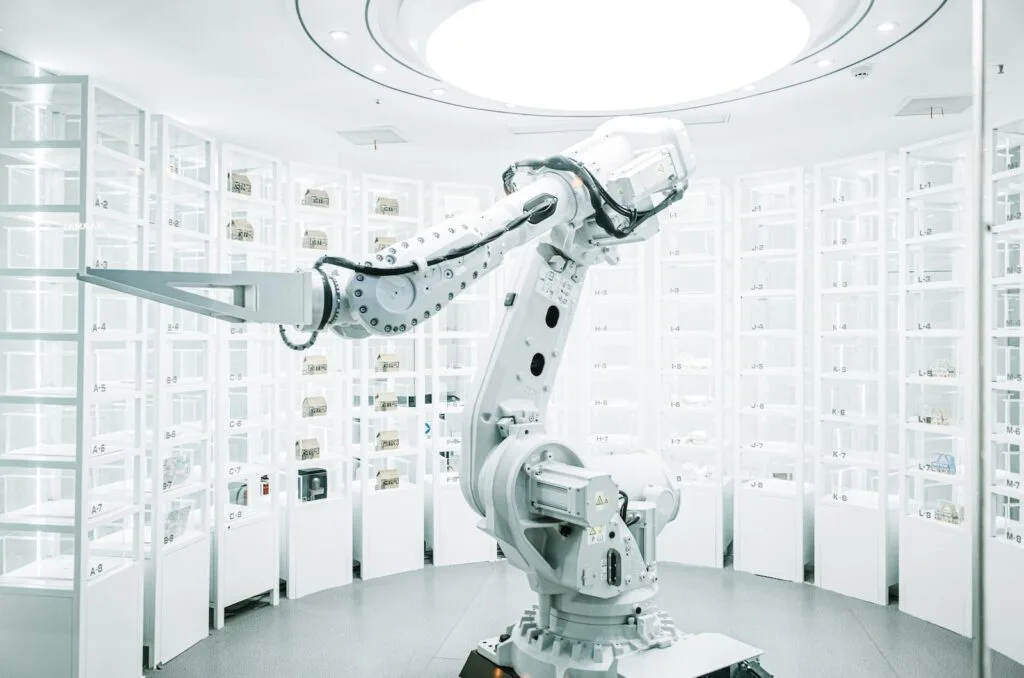
Artificial Intelligence (AI) is changing the way we live and work, and its algorithms are at the forefront of this transformation. These algorithms allow machines to learn from data, adapt to new situations, and make decisions without explicit human intervention. With advancements in machine learning, deep learning, natural language processing, reinforcement learning and evolutionary algorithms; it’s no surprise that AI has become a buzzword across industries. In this blog post, we’ll take a comprehensive look at how artificial intelligence algorithms are revolutionizing our future. So fasten your seat belts as we embark on an exciting journey into the world of AI!
Understanding Artificial Intelligence Algorithms: A Comprehensive Overview

Artificial Intelligence algorithms are the backbone of AI technology. These algorithms allow machines to mimic human intelligence, learn from data, and make decisions based on that learning. Artificial Intelligence Algorithms follow a specific set of rules or instructions to process information and find patterns in it.
Artificial Intelligence Algorithms can be broadly categorized into three types: supervised learning, unsupervised learning, and reinforcement learning. In supervised learning, an algorithm is trained using labeled data where input-output pairs are already known. Unsupervised learning involves training an algorithm with unlabeled data and finding hidden patterns within it. Reinforcement learning uses trial-and-error methods to improve decision-making capabilities by rewarding good behavior.
Artificial Intelligence Algorithms have numerous applications ranging from voice assistants like Siri or Alexa to autonomous vehicles and fraud detection systems used by banks worldwide. Understanding these algorithms is vital for individuals who want to work in this field as well as those who want a better understanding of how AI works at its core.
Artificial Intelligence Algorithms provide machines with human-like intelligence; they are critical components in making AI work seamlessly across various industries today!
The Role of Machine Learning in Artificial Intelligence Algorithms

The role of machine learning in artificial intelligence algorithms cannot be overstated. It is the backbone that enables AI to learn and improve from experience, without being explicitly programmed.
Machine learning involves the use of statistical models and algorithms to enable machines to identify patterns within large datasets. The more data an algorithm has access to, the more accurate its predictions become.
Supervised learning is a popular form of machine learning where algorithms are trained on labeled data sets so they can recognize similar patterns in new unlabeled data. Unsupervised learning, on the other hand, allows machines to find hidden patterns in unlabeled data through clustering or association techniques.
Reinforcement learning is another type of machine learning used for decision-making tasks where an agent learns how to behave by receiving feedback signals from its environment after each action taken, allowing it to adjust and improve its actions over time.
Machine learning plays a crucial role in enabling Artificial Intelligence Algorithms to perform complex tasks such as image recognition, natural language processing (NLP), speech recognition and autonomous driving. As technology continues evolving at breakneck speed, we can expect further advancements that will revolutionize our world even further with AI-powered innovation!
Deep Learning: Unraveling the Potential of Artificial Intelligence Algorithms

Deep learning is a subfield of machine learning that has been revolutionizing the potential applications of artificial intelligence algorithms. Unlike traditional machine learning, which relies on handcrafted features and limited data sets to make predictions, deep learning models can automatically learn complex hierarchical representations from large amounts of unstructured data.
One key advantage of deep learning algorithms is their ability to process raw sensory input such as images, videos, and audio signals directly without manual feature extraction. This allows for more accurate and efficient pattern recognition in a wide range of domains including computer vision, speech recognition, natural language processing, and autonomous systems.
Deep neural networks consist of multiple layers of interconnected nodes that transform the input signal through non-linear functions. The network learns by adjusting the weights between each layer during training using backpropagation algorithm which minimize an objective function like mean squared error or cross-entropy loss.
The success of deep learning lies in its ability to scale with larger volumes and varieties of data from diverse sources such as social media feeds or medical records. Researchers have developed advanced architectures like convolutional neural networks (CNN) for image classification tasks or recurrent neural networks (RNN) for sequential data modeling.
Despite these advancements, there are still technical challenges such as overfitting or vanishing gradients that can limit the performance or generalization ability of deep models. Moreover ethical considerations around privacy protection and bias mitigation must be taken into account when deploying AI systems based on deep learning algorithms in real-world scenarios.
Overall ,deep learning has opened new avenues for developing intelligent machines that can perceive their environments better than ever before; yet it also poses new questions about how we design AI systems that are explainable,predictable,reliable,and fair enough to earn public trust.
Reinforcement Learning: Enhancing Artificial Intelligence Algorithms through Interaction

Reinforcement learning is a type of machine learning where an AI system learns to make decisions by interacting with its environment. It involves the use of trial and error approach, where the algorithm learns from feedback received as it interacts with its surroundings. Reinforcement learning has a wide range of applications in robotics, gaming, and self-driving cars.
One key benefit of reinforcement learning is that it allows machines to learn from experience in real-time rather than relying solely on pre-programmed rules. This makes them more adaptable to changing environments and allows for faster development when compared to traditional programming methods.
The interaction-based approach also enables reinforcement algorithms to optimize their decision-making processes over time. This means that the AI systems can continually improve their ability to perform specific tasks through ongoing interactions with their environment.
In addition, reinforcement learning can lead to breakthroughs in areas such as game theory and strategic decision-making, which have traditionally been difficult areas for computers to excel at due to the complex nature of human behavior.
However, there are still some challenges associated with implementing reinforcement algorithms effectively. These include issues related to scaling up training data sets, selecting appropriate reward functions for different tasks, and addressing concerns around ethical considerations related to using these systems in sensitive domains like healthcare or finance.
Reinforcement learning represents an exciting area of research that has tremendous potential for revolutionizing many industries through enhanced decision making capabilities provided by artificial intelligence algorithms.
Natural Language Processing: Bridging the Gap between Machines and Humans
Natural Language Processing (NLP) is a subfield of Artificial Intelligence that focuses on bridging the gap between machines and humans in terms of communication. It allows computers to process, understand, and generate human language.
One of the main goals of NLP is to enable machines to interpret natural language input from humans accurately. This involves not only understanding the words but also identifying their context and meaning within a sentence or phrase.
NLP technology has numerous applications in different industries such as healthcare, customer service, education, and entertainment. For instance, NLP-powered chatbots can help businesses provide 24/7 customer support by answering frequently asked questions automatically.
Moreover, NLP algorithms are also used in voice recognition software such as Siri and Alexa. These technologies recognize human speech patterns and convert them into text for processing by the device’s operating system.
However, there are still some challenges that need to be addressed when it comes to Natural Language Processing. One key challenge is dealing with ambiguity in language since words can have multiple meanings depending on the context they’re used in.
Nonetheless, research continues towards improving NLP systems further so they can better serve our needs both now and in future scenarios where seamless communication between humans and machines will become increasingly crucial.
Evolutionary Algorithms: Adapting AI for Dynamic Environments
Evolutionary algorithms (EA) are a subset of AI that draw inspiration from the process of natural selection. These algorithms can help machines evolve and adapt to dynamic environments, making them an essential tool in various industries.
The concept behind EA is simple; it mimics the way living organisms develop over time by selecting for desirable traits. The algorithm starts with a population of solutions and evaluates their fitness based on specific criteria. Those with higher fitness are more likely to be selected for reproduction, while those with lower fitness will be discarded.
One significant advantage of EA is its ability to optimize complex problems that traditional methods cannot solve efficiently. For instance, these algorithms can improve supply chain management by predicting how demand patterns might change over time and identifying potential risks before they occur.
EA has also shown promise in cybersecurity where it helps identify suspicious behavior or anomalies within large datasets, thereby preventing cyberattacks before they happen.
However, one major challenge facing evolutionary algorithms is ensuring ethical considerations when using them as decision-making tools concerning human lives or wellbeing. As such, there’s a need to establish ethical guidelines and regulations governing their use in different fields.
Evolutionary algorithms have enormous potential in adapting AI for dynamic environments while presenting unique challenges that must be addressed responsibly.
Overcoming Challenges: Ethics and Bias in Artificial Intelligence Algorithms
As with any technological advancement, there are always ethical considerations to be made. This is especially true for artificial intelligence algorithms that have the potential to make decisions without human intervention. Bias is also a significant concern when it comes to Artificial Intelligence Algorithms.
One of the biggest challenges in creating ethical Artificial Intelligence Algorithms is ensuring they align with our values and beliefs as humans. These values must be clearly defined so that we can avoid creating machines that act against them. Additionally, any biases present within the data used to train these algorithms need to be identified and corrected.
Bias can arise from many sources, including cultural norms or historical events that have shaped our understanding of certain groups of people. It’s important not only to identify where these biases come from but also how they manifest themselves within an algorithm’s decision-making process.
Another challenge is transparency – we need to know how an algorithm arrives at its decisions so that we understand if it aligns with our values and doesn’t perpetuate bias. Accountability should also be built-in so that those responsible for creating or implementing Artificial Intelligence Algorithms can be held accountable if something goes wrong.
Ultimately, overcoming ethical challenges and eliminating bias in AI will require collaboration between technologists, social scientists, policymakers, and ethicists alike. By working together, we can create a future where AI serves humanity ethically and responsibly instead of posing risks through unethical use or malfunctioning due to biased programming.
The Future of Artificial Intelligence Algorithms: Advancements and Possibilities
As we have seen, the advancements in Artificial Intelligence algorithms are rapidly changing the way we interact with technology. From machine learning to deep learning and natural language processing, Artificial Intelligence Algorithms are revolutionizing various industries such as healthcare, finance, transportation, and more.
The future of AI is exciting and full of possibilities. With advancements in quantum computing and data analysis techniques, we can expect even smarter machines that can learn faster than ever before. We may see AI systems that are capable of developing empathy or understanding human emotion better.
There will be continued efforts towards ensuring ethical considerations when creating Artificial Intelligence Algorithms to prevent bias or unintended consequences. The development of explainable AI (XAI) technologies will make it easier for people to understand how an algorithm arrived at a particular decision or recommendation.
In summary, the future of Artificial Intelligence Algorithms is bright with endless possibilities yet to be explored fully. As technology continues to advance further, there’s no telling what breakthroughs might bring about new opportunities for improved performance capabilities across all sectors globally.
[ad_2]
Source link